CLOSER CITIES aims to create a bridge between urban science and urban practice. By collecting cases on urban practice, analysing them on the shoulders of urban science and sharing research outcomes, urban knowledge becomes shareable. In the ‘5 questions’ series, we ask scientists to briefly reflect on their research and the shareability of their insights and projects.
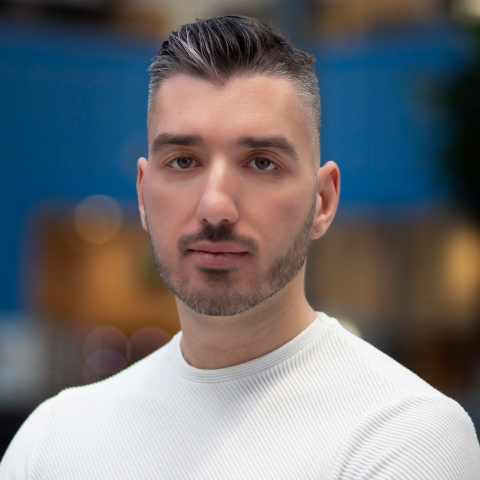
1. What is the main focus of your research (topic, theme, region)?
Location intelligence and spatial analysis
2. Can you give a brief description of your research?
Leveraging on my multidisciplinary background in architectural engineering, spatial planning, and data science, I am passionate about problems at the intersection of machine learning, design, and urban/regional science. I focus on developing computational methods and location-based tools and services to help various stakeholders (e.g. urban planners, policy makers, designers, citizens) make sense of and characterize places and people’s activities, experiences, and interactions. I further seek to understand what processes drive people's behavior to design solutions that improve wellbeing. My methodological approaches are driven by data and informed by theory. I am motivated by how the steadily expanding location ecosystem, fueled by the increasing variety of location data and the advances in technology backing location analytics, can help us better understand human dynamics and potentially improve urban living.
3. How much influence does ‘local context’ have in your field of work? Can results or solutions from your research be shared with other regions easily?
Location, place, and context are core concepts in my research work. They drive the methods that I develop and contribute to designing tools, systems, and services that are location- and context-aware. Examples of such context-related factors include the profile of users, their preferences, emotion status, interactions with other users, the characteristics of the (urban) settings in which these interactions take place, the time of the day and the season, and factors relating to the social and built fabric of cities. I derive information on these factors through a large variety of location-based data sources. The combination of several contextual factors enables me to identify local profiles (e.g. streets that share similar characteristics in terms of structure, land use, streetscape elements, and mobility patterns) that could be shared across neighborhoods in different cities. This facilitates the generalizability of my research results and tool-based solutions, which can be applied to different regions.
4. What are the main lessons learned that can be used by urban initiatives?
The importance of (location-based) data in decision making has been vital in the recent years, and has become even more evident with the current pandemic.
5. How do you think cities can implement these lessons?
I believe that all city stakeholders should be empowered with context-aware tools that support decision making at the local level, so that interventions can be designed with complete knowledge of the context within which they take place. An example of how we could make this happen is the Social Distancing Dashboard (https://bit.ly/social-distancing-dashboard), which I developed together with my team at TU Delft and AMS Institute.